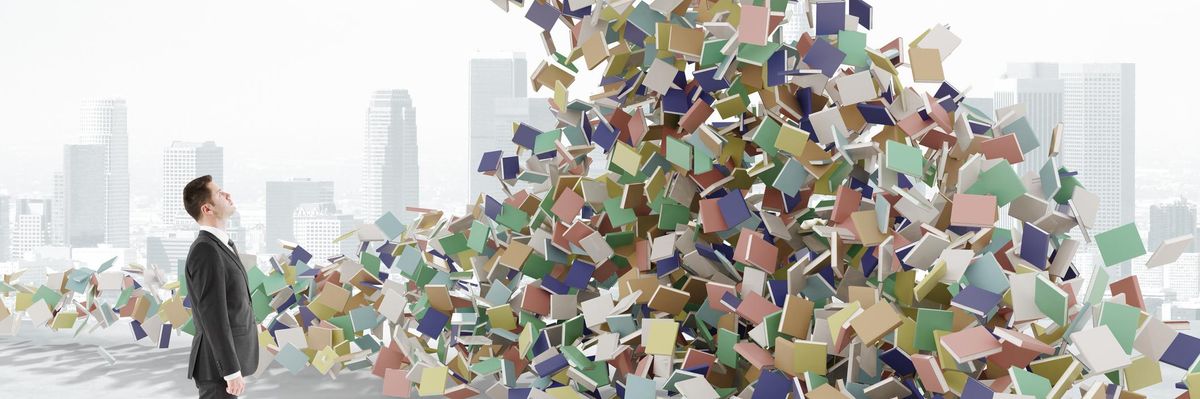
Firstly, many new data concepts have emerged in the last few years, such as data mesh and data fabric (the subject of a future post) which seek to solve the problem that data need to be distributed to the entire organization and users want to access it faster. The idea that we need a more integrated and distributed data environment is well accepted and makes sense in data analytic circles.
The data mesh as defined, in this case by Wikipedia, which for data mesh is as good a source as any: a sociotechnical approach to build a decentralized data architecture by leveraging a domain-oriented, self-serve design. With data mesh, the responsibility for analytical data is shifted from the central data team to the domain teams, supported by a data platform team that provides a domain-agnostic data platform.
To achieve the promise of distributing data to drive insight presumes that the data is of quality and that business domains have the readiness and maturity/skills to harness the power of the data and to "self-serve" to create insights to drive business impact. The vision of distributing data and insights to increase business impact is one that most CDAOs, CAOs, and CDOs embrace; in fact, most of us advocate for centralization first to stabilize and to quality assure data and to create platforms with gold standard data only to then create a hybrid model where the platforms are well maintained but access and teams are decentralized/linked throughout and to the business lines.
A few observations include the fact that it appears that data mesh has been put forward as a conceptual or theoretical idea without defining it well and pointing out its strengths and weaknesses. There is a history in technology circles of failed adoption of CRM platforms and more, so as we journey into this, we don't want to “build it, and they will come” or go from data mesh to data mess. Ok, so let's define the data mesh from what is known so far. Hopefully, we can debunk some of the nebulous shiny object syndromes related to the mesh so we can go forward with our eyes wide open asking good questions and adopting the best parts of the mesh wherever possible.
First and foremost, and I will say this throughout this piece, it will be necessary to put forward a tested commercially viable data mesh solution, which does not exist to date. Well, that's the spirit of test and learn, I suppose. Ok, so here we go. Are you ready to fasten your seat belts? If I could bring back Janice (OMG lady) from the series Friends right now, I would.
The Data Mesh Is A Theoretical Concept Or Construct Which Says The Following...
Bigstock
- Data mesh is a philosophy or a theory to drive architectures. I have not yet seen how this architecture manifests in a transparent way.
- Data is a strategic asset. Ok, no issue with that premise.
- There is no technological solution prescribed for the data mesh as of yet. This could be problematic as data mesh is not a tested construct, especially across industries.
- Data can be self-describing. The idea that data can be discovered and understood in the product sense can be problematic in some industries as it presumes that the business users know and understand the data and can back up the data engineers and analysts in a centralized platform team. I can buy this one if you are in a Silicon Valley software company, but not if you are in banking or financial services, where some product managers don't even have advanced excel skills. The end user maturity is still evolving. Data mesh advocates should define dependencies.
- Provisioned for access. Ok, I can buy this part, but just because you can supply data doesn't mean that the end users understand the data and know how to use it.
- FAIR data: findable, accessible, interoperable, and reusable. Ok, it certainly sounds good if it works smoothly. However, if it results in tons of duplication and the data isn't well defined as promised, what we were trying to solve with data mesh may cause the "Wild West Data Effect (WWDE)" with data replicated and flying around the organization. It is easy to say oh, go ahead and duplicate the data but shouldn't it be planned duplication? Does duplicated data exist in the mesh-o-verse forever?
- Some experts use the term knowledge graph interchangeably with data mesh. No issue with this, but I prefer a well-defined technology solution.
- Whether or not data mesh (DM) is an authentic architecture remains to be seen.
- DM assumes centralized database structures/teams don't work. Not sure I agree that centralized teams and platforms don't work; I think it is more about how CDAOs and CDOs link the team through the operating model and governance through partnerships.
- Data pipelines are fragile. I agree they are and are difficult to manage. Many new tools should be discussed in the context of data mesh which most vendors don't discuss. Where is the discussion of RPA, Pega, Immuta, Matillion, and more?
- Data engineers in the COE for data don't know the data well as they aren't using it. My POV is that it depends on the talent architecture and if it considers experiences and industry. The vendor's statement is an over-generalization that needs to be revisited.
- Analytical data is different from operational data. This point I agree with. But not all data needs to be returned to the data warehouse or data lake. It depends on what you want to do and where you want to do it. Many source systems have operational reporting for operation data, and many also have dashboards. So, this goes back to defining use cases and having a blueprint/strategy for what you want to do and where. I believe some of the vendor commentaries around this point need to be analyzed, and firms need to go back to basics lately and probe on data mesh vendor roadmaps and completeness of vision. What parts of the DM actually exist in any ecosystem?
- There are many monolithic and centralized data repositories. I don't think many firms have even gotten to ETL, especially not globally, let alone ELT and data mesh; much of the dialogue deals with Fortune 50 companies and not even Fortune 1000 companies.
- Data mesh seems to lessen the fact that data analytics is professional competency. It is believed DA is a bottleneck and is not connected to execution, which in most cases is far from the case. If the skill sets genuinely existed in the business lines, this would have happened by now. So we need to examine all of the connected roles in IT and operations to really understand the full picture of bottlenecks and centralize versus decentralize.
- Domain-driven data ownership architecture: I agree with this point if the domains via data stewards can drive their architecture, but I have not seen this often. Domains are often familiar but have no idea how to create data products or do analytics, let alone data modeling. I chuckle when I hear simple comments like "let's change the paradigm." I wish we could have a world where everyone knew analytics and engineering. That would genuinely be nirvana.
- Data as a product (data domains are the product). This is a great idea, but how do we connect these products across all the data as we still want to be customers centric? As long as this doesn't create data product silos, then fine. Most vendors who talk about data products don't' think about enterprise or customer centricity. Having data mesh advocates and researchers explain how to connect customer data to product data and cross multiple domains (LOB data areas) would be good. Using the word data product could be very confusing to business users as we have been talking about customer views for a long time. This needs to be better defined than I have seen in the business press.
- Data should be served and useable at the source. It sounds great. I would like to see how this will work without recreating the processes/tools in DA COEs. I would love to see how vendors push these capabilities upstream to the source. I agree that this would be a significant step change when and if this is technically possible and domains/product owners have the skills to manage this.
- Data moves around, and we can't get to one source of truth. I agree that it has been an elusive goal and only partially achieved (it's more mature in the marketing domain). I would love to understand how vendors who are commenting on data pipelines are coming up with an architecture to make the internal implementation of domains and domain-oriented distribution a reality.
- We don't need the data catalog to have usable data. Alternatives?
- Too many misunderstood terminologies, such as metadata. In the data mesh, the metadata layer still exists. However, DM advocates suggest using simple English and less jargon to describe terms like metadata, master data, catalog, etc. Amen to this one; I agree, but you still need to meet the parameters of what metadata provides.
- The data engineering team still sets up the infrastructure. Yes, they will need to, but data mesh seems to accuse data engineers of holding the business back from using the data, and I disagree with this idea. This depends on the org and engagement models and governance.
- Domain teams in the business can put their data into the lake themselves. I look forward to this day.
- Decentralize storage with centralized infrastructure. How will data governance, policies, and controls work in this DA environment?
- From specialists to generalists. This will require a massive push in training and education. This will work better in tech companies. I would love business and domain users to have the statistical and technical skills to create data products. This change will require new jobs, families, education, and training with significant investment. Also, academic institutions are not currently up to speed on these bleeding-edge ideas to provide a training source and talent pool. Vendors and firms will need to develop their curriculum and training,
- Responsibility for quality and security shifts back to the business lines under the data mesh. It will be interesting to see how the data mesh assures standards and defines security and quality aspects going forward. I agree with this trend as an extension of the data steward concept already in progress under data governance.
In summary, if we are serious about the data mesh, we need to do an entirely new business case and rationalize all of the global concerns that the data mesh presents. For me, data mesh is currently a theory that could turn into an official architecture or a guiding principle. As of now, the data mesh has raised more questions than answers. The data mesh does not necessarily point out the differences and uses case between operational and analytical data, which in my mind, still have a different fit for purpose use case. Changing everyone's mind will take more than just one vendor coining a term to flip the current paradigms on their head without significantly more research and testing. Said differently, we need data about the data mesh (case studies, success stories, and more).
I look forward to your thoughts and comments. What has your experience to date been with the data mesh and how far away do you think you are from adopting this concept?
- How To Organize Data Governance For Business Impact - Work It ... ›
- Does Data Monetization Really Require A Process Discipline ... ›
- The Important Steps Toward Achieving Data Governance - Work It ... ›
- Chief Data Analytics Officer (CDAO): From Mindsets To Skills - Work ... ›
- How To Make The Shift To Data Analytics Literacy - Work It Daily ›
- Data Strategy Is More Important Than Ever In An Age Of AI - Work It ... ›
- Top 5 Questions To Answer To Get Your Data Strategy Right - Work ... ›
- Data Never Sleeps. How Actionable Is Your Reporting? - Work It Daily ›
- Why Chief Data Officers (CDOs) Don't Have More Impact - Work It ... ›
- Data Fabric: What It Is & What We Know So Far - Work It Daily ›
- What To Consider Before You Add A CDP To Your MarTech Stack - Work It Daily ›
- Actionable Reporting (And AI) In A Data-Driven World ›