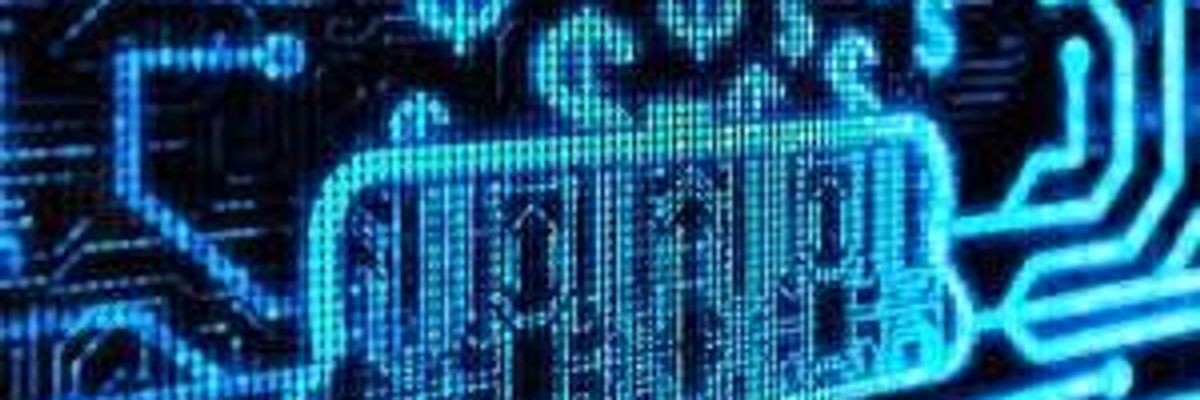
To get good at it, our answer is yes...
Updated this article to include design principles and other elements, as CDOs and CDAOs continue to prove the value of data analytics to COOs and CEOs. The path to the C-suite is in proving the ROI of data analytics, setting up the tests, and providing the evidence.
As an analytics practitioner and leader, I have always stressed the need to create a predictable, repeatable, stable process for analytical data innovation. A data innovation process would help the firm monetize its critical data assets. A monetization capability has applications for several marketing and business domains such as campaign management, customer experience, product development, customer and prospect segmentation, and more (risk, etc.). A good data monetization process has at its core the ability to generate pilots every quarter to facilitate more significant tests and learning. Analytical data innovation has a direct impact on customer satisfaction and retention.
This article was written a few years back initially; however, at that time, the only thing we seemed to hear about was quick wins and not about setting up monetization and test and learn as a process. I posit that the continued focus on quick wins only and not creating a process for learning may be hindering analytical adoption and moving data analytics maturity to the next level. There are good quick wins and bad quick wins depending on whether they are measurable and scalable. It might now be time to suggest a more defined team and process be stood up within the CDAO and design worlds to hit the accelerator on monetizations. In some firms, particularly in Fintech, design practices joined at the hip with CDAOs are beginning to create such a process. My updates to the process are below.
Data Innovation Process Updates
Bigstock
The challenges in developing the analytical data innovation process include:
- Building out a robust data monetization capability to drive consumer and commercial strategy by improving revenue and profit impacts across various activities.
- Developing a strategic analytics roadmap to take the company to the next level. As a starting point, one needs to understand the current state of data analytics and decision sciences.
- Optimizing the function to drive organic growth by retrofitting existing capabilities as the business transforms from offline to digital channels.
- Building a highly regarded, expert team with new skill sets to create analytical products and monetize the data while ensuring compliance with consumer privacy and other regulatory considerations. This includes design skill sets.
- Using Agile and Agile at Scale to create squads and new teams, with new departments coming online, such as design practices and data analytics COEs. Set up lean start-up protocols using design principles to create tests based on a monetization process that has a clear path to be deployed within the company's strategy. The discipline of design can help CDAOs and CDOs bring their quick wins to life. Business cases and ROI goals should be set for each test and monetization.
- Establishing an orientation to innovation and change leadership, focusing on an efficient test and learning discipline to improve the bottom line. In many firms, this is still not a defined process and team, although, as mentioned, start-ups and fintech seem to be ahead in this area.
For each pilot, a monetization process needs to be followed, starting with defining the business case and vetting it with business partners and executives. Is the business willing to make changes and deploy the learnings? After gaining concurrence from the business partners, assemble the right team to keep the monetization effort on track, including executive sponsors, consultants/analytics experts, academics, design practice experts, prototyping firms, line of business sponsors, and IT.
To create the proper pilot development process, you can borrow from both Agile and SDLC, which ensures the formulation of the appropriate business and data requirements. Take the best elements from different project management methods to create the criteria. For example, a development process method can ensure success measures are established for the project.
The following steps are to perform feasibility analysis, create a prototype, and validate the pilot. For most analytical pilots, this involved creating test segmentations or models which can then be deployed in the market so one can read and validate the results. Some of the steps I have gone through in validating pilots included ensuring that we had actual data and systems to deploy the analytic solution. For example, some models require unique data sources to be able to score and implement them at their point of use.
The final step is to determine how far along the monetization cycle you will take an analytical pilot. For example, many times in segmentation, you may find a profitable segment but the segment cannot quickly grow or scale. On the other hand, a pilot that goes into implementation will require execution support and the establishment of success metrics. One new comment on this is that the pilot should have a definition of done and clear success metrics that the CEO and COO are aware of and endorses so that when the pilot is a success, it can be rolled out and celebrated.
My philosophy is to maintain a pipeline of analytical pilots, knowing that only 10%-20% will be fully monetized. This is the central premise behind the test that learns and fails fast and cheaply. Design practices can now help expand the number of pilots that are monetized. See Randy Bean's book Fail Fast Learn Faster for perspectives on this.
Ideally, there is enough diversity of pilots in the queue to generate new analytical products quarterly. This process is iterative as the pilots should be viewed as living solutions, and permanent departments, squads, and tribes should be set up to perpetuate this model.
Given that many CDAOs are still struggling with tenure and investment and proving the ROI, I want your thoughts on how this process can help and what you think is the state of the state is on test and learn and monetization. Are quick wins still quick, or does this process need to usurp quick wins?
- The Biggest Challenge To Cross-Border Cloud Implementation ... ›
- How Nike's Data & Analytics Experts Are Changing the Shoe Business ›
- 3 Ways To Present Quantifiable Information On A Resume - Work It ... ›
- How To Get Management Feeling Safe With Data In The Cloud ... ›
- How To Hire The Best Data Analytics Leaders - Work It Daily ›
- How To Ensure The Data Mesh Doesn’t Create A Data Mess - Work It Daily ›
- The Data Science ROI Of Moving To The Data Lakehouse - Work It Daily ›