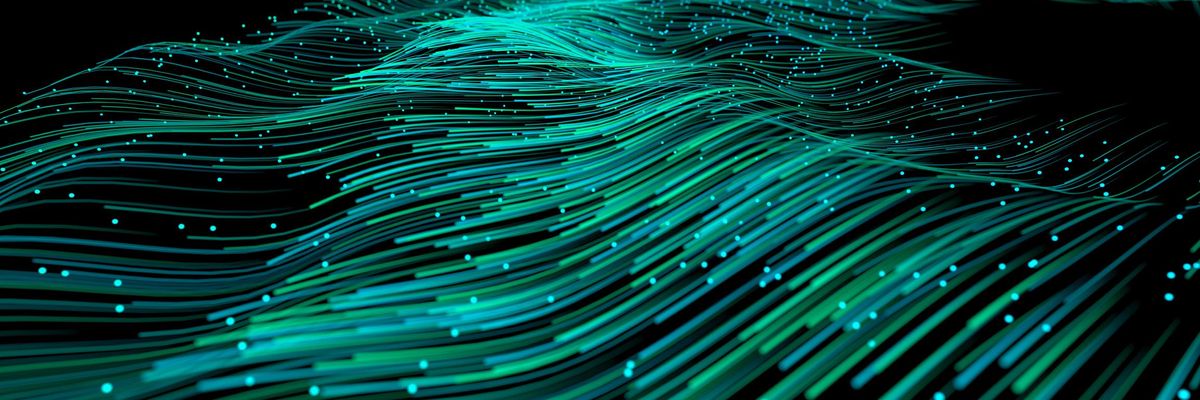
Earlier in my career, as a data analyst, the biggest area of frustration for my marketing clients was the lag time between their requests and the business insight. The lag was extended if the required data had to be ingested from a new source. The old ETL framework and associated data management tasks created long waits for impactful insights to reach marketing tactics.
Over the course of my career, despite advances in data strategies and infrastructure, similar questions remain:
- “Why can’t I get my analytics sooner?”
- “Why can’t I access my own data?”
- “Why does it take so long to source new data that we need for analytics?”
A recent concept that seeks to relieve the stress associated with these questions is the data fabric. According to Gartner, a data fabric is...
“An architecture pattern that informs and automates the design, integration, and deployment of data objects regardless of deployment platforms and architectural approaches.
"It utilizes continuous analytics and AI/ML over all metadata assets to provide actionable insights and recommendations on data management, integration design, and deployment patterns.
"This results in faster, informed, and (in some cases) complete automation of access and sharing.”
Quite a long multi-layer definition, but based on this promise of data fabrics, data analytics teams can now:
- Avoid having to move data
- Have better access controls and democratize access
- Provide faster, more automated analytics through AI
Business managers often think about these issues and their impact on their business:
Speedier time to insight (shorter cycle times): No more ETL, or ELT, or other time-intensive data management tasks associated with data analytics. AI/ML tools will automate data tasks and data virtualization will allow analysts to go directly to the source elements avoiding time-intensive access and exploration—with the exciting possibility of eliminating extract tools, schedulers, and more.
Expense savings: By going directly to the source, no intermediary platforms, systems, or repositories will be needed. Therefore platform/tool expenses and recurrent maintenance expenses would be saved.
Why Move Data? (When You Don’t Have To!)
Bigstock
The problem many firms face is the storage of their data in many silos and systems—clouds (lakehouses), warehouses, source systems, ODSs, CRM systems, marketing marts, and in some cases even legacy SAS files. Each of these silos introduce workflows that are necessary but increase time-to-insight. How can organizations use all their data efficiently?
The idea behind the data fabric is to break down data silos and get data into the hands of the users. The data fabric is a tapestry connecting data across all platforms to users, creating efficiencies by not having to move data. Data automation capabilities within the fabric further drive efficiencies by providing accessible, quality data for use by AI and other tasks.
One of the key enablers of a data fabric is a virtualization layer that provisions data needs directly (from transaction and operational systems) without moving or copying data. Data tasks like sourcing, extracting, cleaning/transforming, etc. are all automated. The fabric also helps manage the lifecycle of data, for example, by governing your data using active metadata to enforce the policies, including access, compliance, and quality.
In summary, data fabrics are still evolving—the architecture has merit, the concept seems more developed than that of the data mesh (which is much more of a theory), and foundational tech capabilities are well-enabled. I see fabrics as having great promise, but more as an architecture strategy with a focus on less data movement, fewer silos, more access to data, and faster analytics. Will fabrics eliminate the need to move data completely? I don’t think so (at least in the near term), as there will always be a need to move and replicate some data. Would love to hear from the vendors in the space on this post as well.
Is it data fabric or data fabrication? Only further development (and time) will tell, but I’m betting on the fabric’s promise!
I’m also betting on you, my readers—I bet that a discussion with you on this topic will surface many challenges and opportunities for data fabrics. What would you like to learn more about? What has been your experience with the data fabric? Is it fabric or fabrication? Please reply to this post with your comments so we can all dive deeper into the strategic, tactical, and business implications of this fascinating area.